Assessing Health Impacts of Pictorial Health Warning Labels on Cigarette Packs in Korea Using DYNAMO-HIA
Article information
Abstract
Objectives
This study aimed to predict the 10-year impacts of the introduction of pictorial warning labels (PWLs) on cigarette packaging in 2016 in Korea for adults using DYNAMO-HIA.
Methods
In total, four scenarios were constructed to better understand the potential health impacts of PWLs: two for PWLs and the other two for a hypothetical cigarette tax increase. In both policies, an optimistic and a conservative scenario were constructed. The reference scenario assumed the 2015 smoking rate would remain the same. Demographic data and epidemiological data were obtained from various sources. Differences in the predicted smoking prevalence and prevalence, incidence, and mortality from diseases were compared between the reference scenario and the four policy scenarios.
Results
It was predicted that the optimistic PWLs scenario (PWO) would lower the smoking rate by 4.79% in males and 0.66% in females compared to the reference scenario in 2017. However, the impact on the reduction of the smoking rate was expected to diminish over time. PWO will prevent 85 238 cases of diabetes, 67 948 of chronic obstructive pulmonary disease, 31 526 of ischemic heart disease, 21 036 of lung cancer, and 3972 prevalent cases of oral cancer in total over the 10-year span due to the reductions in smoking prevalence. The impacts of PWO are expected to be between the impact of the optimistic and the conservative cigarette tax increase scenarios. The results were sensitive to the transition probability of smoking status.
Conclusions
The introduction of PWLs in 2016 in Korea is expected reduce smoking prevalence and disease cases for the next 10 years, but regular replacements of PWLs are needed for persistent impacts.
INTRODUCTION
Although, through several tobacco tax increases and various non-price anti-smoking measures, the smoking rate of Korean males was reduced to 43.3% in 2015 from 52.2% in 2006, it is still the second highest among the countries affiliated with the Organization of Economic Cooperation and Development (OECD) [1]. Recognizing this high smoking rate, the Republic of Korea (hereafter Korea) increased its cigarette tax in January 2015 and introduced pictorial warning labels (PWLs) on tobacco packages in December 2016. Further decreases in the smoking rate are expected through these policy implementations.
In Korea, pictorial warnings and text warnings are now required to cover 30 and 20% of each of the front and the back side of a cigarette pack [2]. This is the first time in its history that Korea introduced PWLs following the recommendations in Article 11 of the WHO’s Framework Convention for Tobacco Control (FCTC). Currently, 10 pictures are distributed, and these will be replaced with a new set every 24 months [2]. Along with pictorial warnings, much stronger text warnings (e.g., accompanying a photo of lung cancer: “The cause of lung cancer. Will you still smoke?”) replaced the old text warnings (e.g., “Smoking is a cause of diseases like lung cancer. It is difficult to quit once you start smoking.”) [2]. In order to ensure this new policy results in intended outcomes such as reduced smoking prevalence and smoking-related diseases and deaths, the government needs to monitor these outcomes. However, before monitoring, we must determine the expected levels of these outcomes in order to evaluate whether the observed outcomes are satisfactory or not.
Health impact assessment (HIA) is a combination of processes, methods, and tools that enables us to assess the potential impacts of a policy, a program, or a plan for population health [3]. HIA is usually conducted before a policy, a program, or a plan is implemented, but it can also be conducted concurrently. A concurrent HIA, however, involves monitoring an intervention and is useful when the nature and severity of health impacts are uncertain [4]. The recommendations from a concurrent HIA are intended to influence decision makers as a policy progresses [4]. A concurrent HIA is necessary in Korea in that although it is known that PWLs will lower smoking prevalence, there are uncertainties about the nature and the strength of the change as well as other health impacts from introducing PWLs.
The two key elements of HIA are to predict the impacts of different policy alternatives on health and to present these predicted impacts for consideration in decision making [5]. Although quantification is often required in decision making, a quantitative approach has not been widely used in HIA [6]. In Korea, HIA in environmental impact assessment takes the quantitative approach. However, none of the 14 HIAs conducted in the public health field in Korea have quantified potential health impacts [7]. Although a qualitative approach to HIA has been beneficial in supporting decision making [7], there is a need to utilize a quantitative tool for HIA, particularly for public health policies in Korea.
Because PWLs were recently implemented, Korea needs a concurrent HIA in order to monitor and evaluate the effectiveness of PWLs and should use a quantitative tool. To this end, this study aimed to predict the 10-year health impacts of the implementation of PWLs in 2016 in terms of smoking prevalence, disease prevalence, and mortality using a standard HIA quantification tool, DYNAMO-HIA. The nature and the strengths of the impacts of the new anti-smoking policy found in this study may be helpful for current and future decision making on PWL policies.
METHODS
DYNAMO-HIA
DYNAMO-HIA is a dynamic simulation tool using modeling based on Markov [8]. It was developed to quantify exposures to health risks and to estimate their health impacts [9]. It is a generic tool that can include other diseases and risk factors beyond the nine diseases—ischemic heart disease (IHD), diabetes, chronic obstructive pulmonary disease (COPD), stroke, lung cancer, breast cancer, esophageal cancer, oral cancer, and colorectal cancer—and the three risk factors—smoking, drinking, and obesity—that are included in the tool by default [8].
DYNAMO-HIA was developed by a group of experts in Europe to respond to the demand for a user-friendly, valid tool for quantification in HIA [9]. DYNAMO-HIA is publicly accessible because it is available online (http://www.dynamo-hia.eu/) for free, and it can produce rich outputs that include disease incidence, prevalence, and mortality as well as summary measures of population health with modest data requirements [9].
DYNAMO-HIA is based on causal epidemiological relationships among a risk factor, incidence and prevalence of disease, and mortality [10]. When a policy intervention alters the exposure to a risk factor, the disease incidence first changes, followed by disease prevalence and mortality. The data needed for DYNAMO-HIA consist of demographic data and epidemiological data. Demographic data should include the population size, future newborns, and all-cause mortality. Epidemiological data should include the incidence, prevalence, and mortality of the diseases pertinent to the HIA, the level of exposure to a risk factor, and the relative risk (RR) of disease occurrence from the risk factor by sex and age [8].
DYNAMO-HIA compares alternatives of policy intervention with business-as-usual in order to estimate the impacts of those alternatives [9]. DYNAMO-HIA assumes that policy alternatives change the prevalence of a risk factor in the first year and/or the transition probability among the states of the risk factor. The prevalences of the risk factor in the following years are determined by the model [10]. The validity of the results produced by DYNAMO-HIA has been found to be satisfactory [8].
Policy Scenarios
In our study, the reference scenario or the business-as-usual scenario was defined as the smoking prevalence in the year previous to the policy implementation, which was 2015 in this case, and was assumed to remain the same for the next 10 years. Table 1 shows the four policy scenarios which were compared to the reference scenario. The main policy scenario was designed as the fact that the introduction of PWLs in 2016 would reduce the smoking rates in 2017. This reduction was the baseline in the model. In order to find a relative strength of health impacts of PWLs, we constructed a hypothetical policy scenario of a cigarette tax increase by 100% in 2016. For each of the two scenarios, a conservative scenario was defined as a smoking reduction of 50% of the optimistic scenario.
The effect size of each policy was obtained from either systematic review articles or reports published by international organizations. First, according to a systematic review on the effect of warnings on tobacco packages [11], warning phrases or pictures may reduce the smoking rate from 0.18 to 0.16, which is equivalent to a 12.50% decrease. This appears to be a reasonable and modest estimate, because Huang et al. [12] recently estimated the relative reduction in smoking prevalence from PWLs to be 12 to 20%, and one Canadian study [13] found a 12% reduction. The effect sizes for the two PWL scenarios (pictorial warnings, the optimistic [PWO] scenario and pictorial warnings, the conservative [PWC] scenario) were defined as 12.5 and 6.25%, respectively.
Second, the World Bank reported that the price elasticity of cigarette demand ranged from –0.15 [14] to –0.20 [15]. Ranson et al. [14] argued that those in their teens and twenties had 3 and 5 times higher price elasticity, respectively, than those in their 30s. In this study, relatively conservative effect sizes from the literature were used. The optimistic cigarette tax scenario (tax increase, the optimistic [TIO] scenario) assumed that the price elasticity of adults and adolescents were -0.15 and -0.45 (3 times -0.15) and the conservative scenario (tax increase, the conservative [TIC] scenario) assumed -0.07 and -0.22 (3 times -0.07) (Table 1).
Data
Population data such as population size, number of future newborns, and all-cause mortality were obtained from the Korea Statistics Office, all for year 2015, since this was the most recent year data were available [16-18]. Epidemiological data needed for DYNAMO-HIA and their sources are listed in Table 2. Six smoking-related diseases were included in this study: IHD, diabetes, COPD, stroke, lung cancer, and oral cancer. These are the diseases that were found to have the largest number of disability-adjusted life years (DALYs) attributable to smoking for the entire population, combining males and females in Korea [19].
The definition of each disease of interest and the source and the year of the epidemiological data, including incidence, prevalence, and RR from smoking for each disease, are presented in Table 2. The incidence of IHD and COPD were not available for the Korean population and thus they were estimated by using DiSMod II [20]. In addition, the RRs of incidence of IHD, stroke, and oral cancer associated with smoking were not available in Korea. In this case, the data from other East Asian countries were used. For example, the RR of IHD and stroke were obtained from China [27,30], COPD from Singapore [28], and oral cancer from Japan [32]. We obtained the incidence and prevalence from the most recent year of data available, which was 2015 in most cases.
The smoking rates for the reference scenario were obtained from the 2015 Korea Nutrition and Health Examination Survey [25]. The smoking rates in the baseline years were calculated according to the effect sizes presented in Table 1. The transition probability between smoking status from one year to the next was estimated from the 2009-2013 Korea Medical Expenditure Panel data for adults (Supplemental Table 1). Smoking status was categorized into current smoker, past smoker, and non-smoker. All the data across 4 years were pooled. The unit of analysis was the smoking status of the two adjacent years ignoring missing data.
Analysis
When applying the effect size of the reduction in smoking rate in each of the policy scenarios, we assumed that the smoking reduction occurred solely among current smokers. In other words, the reduction in smoking rate was assumed to be caused by the increase in past smokers and not by the reduction in new smokers.
DYNAMO-HIA provides raw data for frequencies of groups by smoking status each year as well as the numbers of cases, new cases, and deaths by disease and smoking status each year. In order to obtain descriptive statistics of these data and to estimate the transition probability among smoking status, we analyzed these raw data using Stata version 13.0 (Stata-Corp, College Station, TX, USA).
A sensitivity analysis was performed on transition probability, where we compared the study results with those obtained from the assumption of the alternative net transition probability. Aside from the smoking rate as affected by policy interventions, the transition probability, which determines the way in which future smoking behavior will change, is very important for predicting the future smoking rate. DYNAMO-HIA provides data for the assumption of net transition, which assumes that the prevalence of risk factors by sex and age, as well as the smoking rate in this study, will remain the same in the future [8].
RESULTS
Predicted Reductions in Smoking Rates
Figure 1 shows the results of the differences between the predicted smoking rate of each anti-smoking scenario and that of the reference scenario for those aged 19 and over. Each of the lines represents the predicted smoking rate for one of the scenarios—PWO, PWC, TIO, and TIC—when the smoking rate of the reference scenario was assumed to be zero. For both males and females, the predicted impact on the reduction in smoking rates diminished over time.
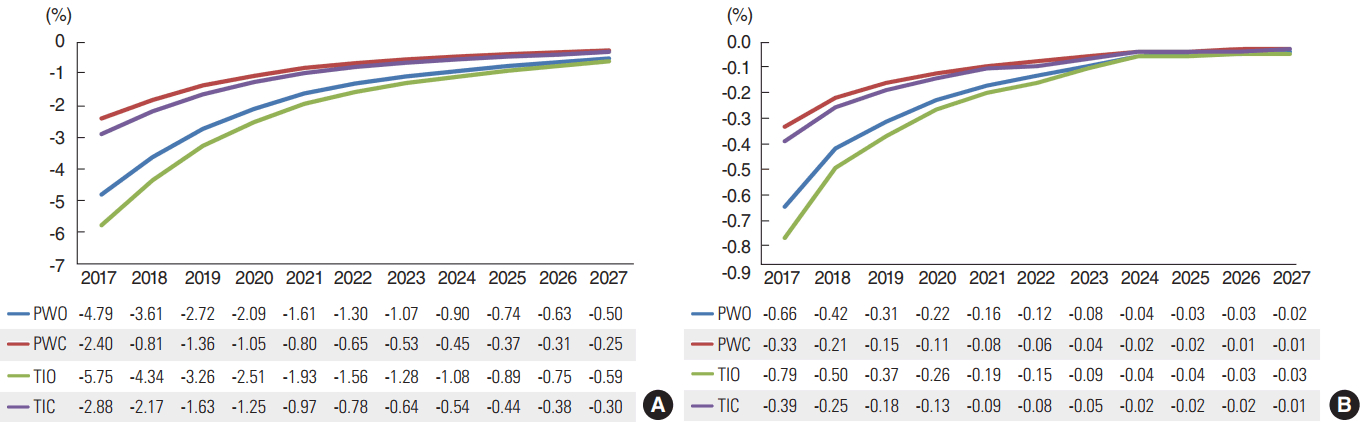
Differences in smoking rates between the four anti-smoking scenarios and the reference scenario (A) males and (B) females. PWO, pictorial warning, the optimistic scenario; PWC, pictorial warning, the conservative scenario; TIO, tax increase, the optimistic scenario; TIC, tax increase, the conservative scenario.
The graph on the left side is for males (Figure 1A). The impact on the smoking rate was the greatest in scenario TIO, followed by PWO, TIC, and PWC. The difference in the smoking rate compared to the reference scenario in 2017 was –5.75% with TIO, -4.79% with PWO, -2.88% with TIC, and –2.40% with PWC, respectively. In 2027, all the predicted smoking rates converged to that of the reference scenario, but the smoking rates associated with each anti-smoking policy remained lower than that of the reference scenario: -0.50% with PWO, -0.25% with PWC, -0.59% with TIO, and -0.30% with TIC, respectively.
The graph on the right side is for females (Figure 1B). As with the males, the greatest predicted reduction in the females’ smoking rate occurred with TIO followed by PWO, TIC, and PWC, and all the anti-smoking policy scenarios showed persistently lower smoking rates than the reference scenario.
Predicted Reductions in Cases, New Cases, and Deaths
Table 3 shows the predictions of cumulative cases, new cases, and deaths for 10 years beginning in 2017 for each anti-smoking policy scenario as compared to the reference scenario. In both males and females, diabetes, COPD, and IHD had the greatest numbers of cases and new cases compared to the reference scenario, followed by stroke, lung cancer, and oral cancer. The sums of the cases and new cases across all 6 diseases were greatest in scenario TIO (-247 024 and -37 844 for males, -31 304 and -4111 for females) and smallest in scenario PWC (-102 928 and -15 769 for males, -13 040 and -1713 for females). Note that these numbers are not the number of real persons, because a person might have multiple diseases. The deaths due to all 6 diseases in males were estimated to be reduced by 135, 67, 161, and 81 for PWO, PWC, TIO, and TIC, respectively.
Figure 2 shows excess numbers of prevalent cases and new cases of diabetes over time as an example. The excess numbers of new cases diminished over time, but the excess numbers of prevalent cases increased over time. The other diseases had the same patterns.

Differences in (A) incidence and (B) prevalence of diabetes between the four anti-smoking scenarios and the reference scenario. PWO, pictorial warning, the optimistic scenario; PWC, pictorial warning, the conservative scenario; TIO, tax increase, the optimistic scenario; TIC, tax increase, the conservative scenario.
Sensitivity of the Model for the Transition Probability of Smoking Status
The graphs in Supplemental Figure 1 show the reductions of smoking rates by anti-smoking scenarios when net transition was assumed. The differences in smoking rates between the anti-smoking policy scenarios and the reference scenario based on the net transition assumption also showed that the impact was greatest in TIO, followed by PWO, TIC, and PWC. In addition, the differences diminished as time approached 2027. However, the slopes of the changes were more gradual and the magnitudes of the impacts remained greater compared to the original transition assumption. Accordingly, the results in Supplemental Table 1 show that the reductions in cases, new cases, and deaths were much greater than those shown in Table 3, which implied that the model was sensitive to transition probability.
DISCUSSION
Since 1995, when the National Health Promotion Act was enacted, Korea has implemented various tobacco control policies to curtail the country’s high smoking prevalence and its negative health consequences. The cigarette tax has been raised 7 times, smoke-free areas have been expanded from public places to worksites and restaurants, and smoking cessation treatment has been covered through National Health Insurance,
with programs including physician interventions, a quitting hotline, and smoking cessation clinics in public health centers [33]. Korea had also been trying to introduce PWLs since Korea ratified the FCTC in 2005, which required the member states to adopt PWLs within 3 years after its ratification. However, it was December 2016 when Korea finally implemented PWLs and became one of the 105 countries that have adopted PWLs [34].
Thus far, research studies on PWLs in Korea have focused on consumer responses, specifically in terms of cognitive and emotional responses [35], and no studies have tried to predict future health impacts of PWLs. This study has predicted the impacts of the PWLs on tobacco packages in Korea in 2016 in terms of smoking rates and prevalence, incidence, and mortality from the 6 most burdensome diseases from smoking for Koreans. The impacts on smoking rates due to PWLs were predicted to remain for the next 10 years, although the effects became less than 1% in males by 2024 and negligible by 2027. White et al. [36] also found that the effect of graphic health warnings on cigarette packs diminished after 5 years. However, the Korean government announced that they would replace the pictorial warnings every 24 months [2]. Therefore, the health impacts in reality might be greater than those found in this study if the policy is indeed implemented as planned.
As a consequence of this reduction in the smoking rate, it was predicted that the incidence and the prevalence of 6 chronic diseases would be also reduced. The reductions in cumulative cases and new cases were greatest for diabetes, COPD, and IHD, followed by stroke, lung cancer, and oral cancer. By 2027, PWLs are predicted to prevent, at maximum, 85 238 cases of diabetes, 67 948 of COPD, 31 526 of IHD, 21 036 of lung cancer, and 3972 of oral cancer in total. A Dutch study also found that the reduction in disease prevalence as compared to the reference scenario was greatest for COPD, followed by IHD and lung cancer, when a population-wide anti-smoking policy was implemented, as in this study [10].
Levy et al. [37] estimated the effects of PWLs in the US on smoking prevalence and smoking-attributable deaths using the US SimSmoke model. They chose a much lower effect size of PWLs (4% for best estimate, 2% for lower bound, and 8% for upper bound) than ours. However, their estimates of health impacts were much larger. Levy et al. [37] estimated that PWLs would reduce smoking prevalence by 5% in a 5-year time span and by 10% in a 50-year time span, whereas the initial 4.79% reduction in smoking prevalence in males from PWLs diminished to 0.59% at 10 years in our study. Moreover, the predicted deaths were 1793 for males and 1050 for females 5 years after the baseline. In our study, deaths averted from PWLs were estimated to be 135 for males and 15 for females over a 10-year period. Even after considering that the population size of the US is about 6 times larger than that of Korea, our estimates were smaller than those of US SimSmoke.
These differences in results may be explained in a few ways. First, RRs of smoking with regard to disease incidence and mortality are smaller in Asians than in the US [38]. Second, only 6 diseases were considered in this study, while SimSmoke considered all smoking-attributed diseases. Third, SimSmoke is different from DYNAMO-HIA in that it uses the potential impact fraction (PIF) to model the transition probability [10]. Models using the PIF approach do not model risk-factor states, which may lead to biased estimates [39]. On the other hand, DYNAMO-HIA models risk-factor states every year by age and sex and then estimates the incidence based on the RR of the risk factor and then the prevalence and mortality.
We also compared the health impacts of PWLs with the hypothetical policy scenario of a cigarette tax increase. This comparative approach was used because DYNAMO-HIA is rather optimal for a comparison of different policy scenarios in terms of the impacts on a population [8]. We found that the health impacts of PWLs were smaller than those from a 100% tax increase and larger than those from a 50% tax increase. Since Korea raised the cigarette tax by 2000 Korean won (about US$2.00), which resulted in an 80% increase in the price of cigarettes, the impacts from the cigarette tax increase in 2015 may be similar to the impacts from PWLs in 2016. There are not many studies comparing the future benefits of PWLs and tax increases, partly because many developed countries had not adopted PWLs until recently [34]. In one study of African countries, Levy et al. [40] found that a tax increase had a greater benefit than PWLs.
This study found that the prediction of future smoking rates and the health impacts of anti-smoking policies were sensitive to the transition probability of smoking status. Even though the order of the magnitudes of the impacts were the same across the four scenarios, the absolute magnitudes of the impacts when net transition was assumed became as much as twice as large as those in the original transition assumption. Therefore, researchers performing future studies using DYNAMO-HIA should take care in choosing the transition probability of a risk factor.
This study has a few limitations. First, this study assumed the reduction of the current smoking rate was caused by an increase in past smokers. However, anti-smoking policies can reduce smoking initiation and hence cause an increase in nonsmokers. Since the risk of disease incidence may differ between past smokers and non-smokers, the simulation results may change if we change the target group of a policy. Second, few input data were obtained from Asian countries due to the lack of local data. As Korea becomes rich in local epidemiological data, the quality of DYNAMO-HIA will also improve. Third, multiple anti-smoking policies are implemented in reality, but only the separate impact of PWLs was estimated in this study. Future studies need to find ways to model multiple policy changes using DYNAMO-HIA. Fourth, this study involved only 6 smoking-related diseases. Future studies need to examine a more comprehensive list of smoking-related diseases including stomach cancer, liver cancer, asthma, etc.
In conclusion, it was predicted that the introduction of PWLs in 2016 in Korea would lower the smoking prevalence both in males and in females for the next 10 years relative to no intervention, and the health impacts in terms of disease prevalence, incidence, and deaths would be less than the impacts of a 100% cigarette tax increase but more than the impacts of a 50% of cigarette tax increase. However, since the impacts will become negligible in a few years, decision makers need to replace the current pictorial warnings every 24 months as planned so that the impacts of PWLs will persist.
ACKNOWLEDGEMENTS
This study was supported by the sabbatical year supporting program in Soonchunhyang University.
Notes
CONFLICT OF INTEREST
The author has no conflicts of interest associated with the material presented in this paper.
SUPPLEMENTAL MATERIAL
Effect of the change in the smoking status transition: impacts of anti-smoking policies by sex
Differences in smoking rates between the four anti-smoking scenarios and the reference scenario when net transition was assumed (A) males, (B) females. PWO, pictorial Warning, the optimistic scenario; PWC, pictorial warning, the conservative scenario; TIO, tax increase, the optimistic scenario; TIC, tax increase, the conservative scenario.